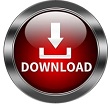
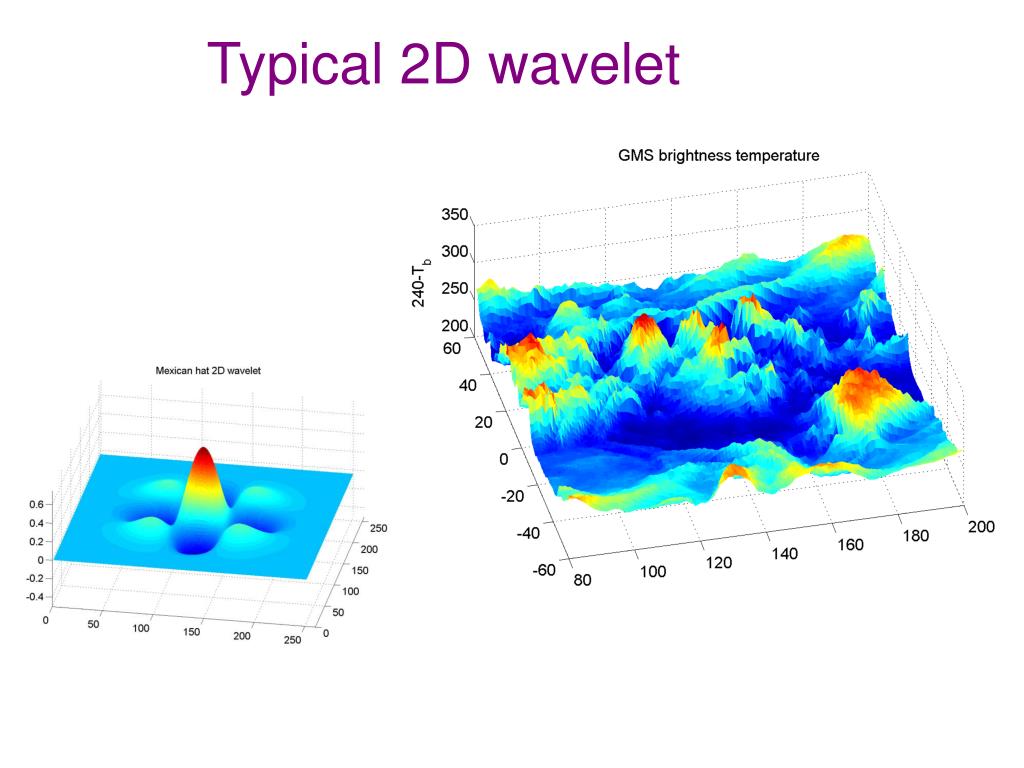
These wavelet-based methods have demonstrated its efficiency in denoising and have achieved state-of-the-art PSNR performances. These methods known as wavelet-based denoising techniques can be viewed also as fixed basis dictionaries to whole images. Denoising is accomplished by transforming back the processed wavelet coefficients into spatial domain. At each scale, some operations, such as thresholding and statistical modeling, can be performed to suppress noise. It decomposes the input signal into multiple scales, which represent different space-frequency components of the original signal. Wavelet transform (WT) has proved to be effective in noise removal. Generally, image denoising methods can be classified as spatial domain methods, transform domain methods, which are introduced in more detail in. To obtain a good estimation image \(\tilde \), image denoising has been well-studied in the field of image processing over the past several years. Owing to solve the clean image x from the (1) is an ill-posed problem, we cannot get the unique solution from the image model with noise. The goal of image denoising is to recover the original image from its noisy observation where the main challenge is to remove noise while retaining as much as possible the important signal features and improving the PSNR as a common metric used to assess the performance of the denoising methods, the higher the value of PSNR, the more accurate is the denoising. Where y is the observed noisy image, x is the original image, and b represents additive zero-mean white and homogeneous Gaussian noise with standard deviation σ.

Mathematically, the problem of image denoising can be modeled as follows: Noise suppression is of great interest in digital image processing, considering that the quality improvement of corrupted images is of essential importance for the majority of image processing areas, including analysis of images, detection of edges, and pattern recognition. Digital images may be contaminated during acquisition, transmission, and compression, by diverse types of noise, generated by different causes, such as signal instabilities, defective sensors, physical deterioration of the materiel due to aging, poor lighting conditions, errors in the transmission due to channel noise, or interference caused by electromagnetic fields. Noise means that the pixels in the image show different intensity values instead of true pixel values. It may arise in the image as effects of basic physics-like photon nature of light or thermal energy of heat inside the image sensors. Noise is a random variation of image intensity and appears as grains in the image. Image denoising as a low-level image processing operator is an important front-end procedure for high-level visual tasks such as object recognition, digital entertainment, and remote sensing imaging.
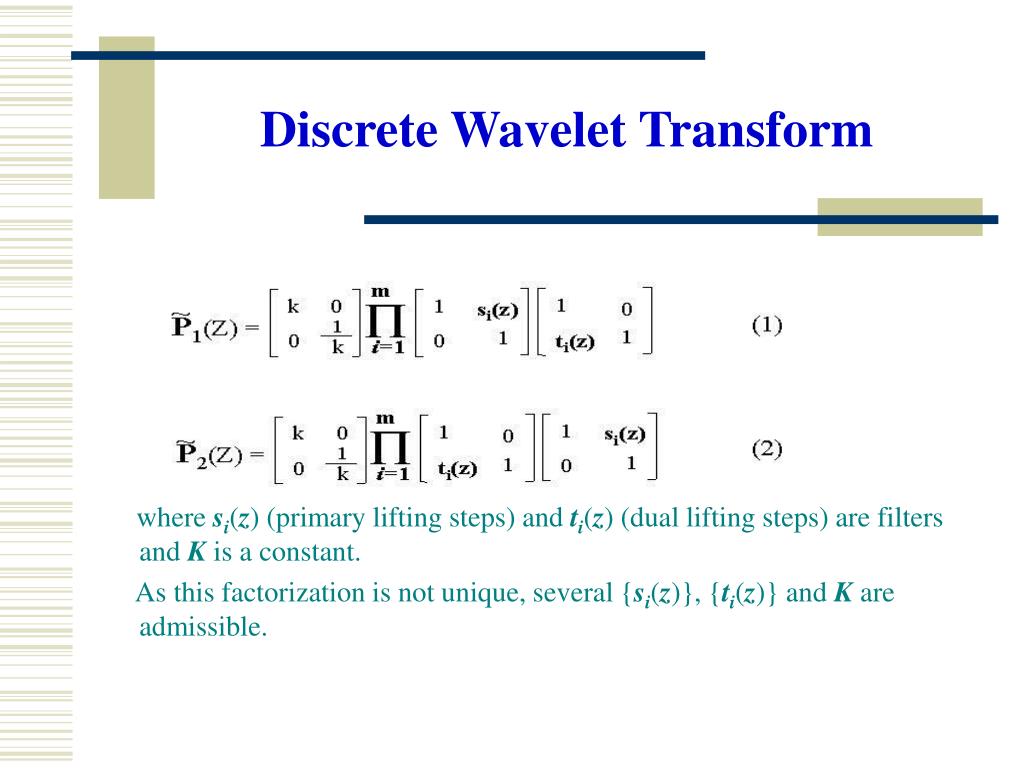
Experimental results on benchmark test images show that our proposed method achieves very competitive denoising performance and outperforms state-of-the-art denoising methods, especially in the peak signal to noise ratio (PSNR), the structural similarity (SSIM) index, and visual effects with different noise levels. Using the K-Singular Value Decomposition (K-SVD) algorithm, we obtain an adaptive dictionary by learning over the wavelet decomposition of the noisy image. The approach taken aims at exploiting the merits of the wavelet transform: sparsity, multi-resolution structure, and similarity with the human visual system, to adapt an unsupervised dictionary learning algorithm for creating a dictionary devoted to noise reduction. In this paper, we propose a novel wavelet denoising approach based on unsupervised learning model. Most of these methods, however, still have difficulties in defining the threshold parameter which can limit their capability. The best currently available wavelet-based denoising methods take advantage of the merits of the wavelet transform. A number of methods have been presented to deal with this practical problem over the past several years. Image denoising plays an important role in image processing, which aims to separate clean images from noisy images.
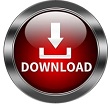